갤러리 보기
Search
1. What is singing voice synthesis?
Singing voice synthesis (SVS) is the task of generating a natural singing voice from a given musical score. With the development of various deep generative models, research on synthesizing high-quality singing voices has been emerging recently. As the performance of the SVS improves, there are increasing cases in which the technology is applied to the production of actual music content.
.png&blockId=eb36e24a-94b0-47c3-bd3f-a538558d3466)
2. Challenges
There are various challenges to designing a singing synthesis system that can freely generate high-quality, natural-sounding singing voices.
•
First of all, the problem is that it is difficult to construct a dataset. In general, since singing is difficult to be released due to issues such as copyright, we have limitations in collecting public singing datasets. It is also difficult to access clean singing voices, as many are released with accompaniment. Lastly, singing synthesis requires not only a clean singing voice but also appropriate sheet music information corresponding to it, and the process of annotating it is time-consuming and costly.
•
In order to respond to this problem, 1) research on effectively modeling singing using only small data (LiteSing, Sinsy), 2) research on securing data sets using technologies such as sound source separation and automatic notation from various sound sources existing on the online web (DeepSinger), 3) Research on creating and presenting singing datasets free from copyright issues such as nursery rhymes is being conducted (CSD).
•
In our lab, we collect 200 songs and conduct research using them. First, we purchase an accompaniment MIDI file for K-POP music from a MIDI accompaniment producer, then hire an amateur singer to sing and record the song to the accompaniment. Later, by manually correcting minute differences in timing and pitch between the actual singing and the melody MIDI, the song and score pair data are obtained. Using this data, we are working on singing voice synthesis modeling while at the same time trying to obtain more sophisticated annotations through transcription and alignment studies.
Dataset example (audio, midi stereo)
2.2 Sound quality
•
With advances in speech synthesis studies, generating results of adequate quality in speech synthesis has advanced a lot. However, for singing synthesis technology to be used in real industry, studio quality results are required. Therefore, we are exploring different methods aimed at generating a 44 kHz sound source.
•
Unlike speech, singing voice 1) has a wide pitch range, 2) contains many notes with long duration, and 3) it is necessary to model a high sampling rate. We are trying to solve this problem based on the latest research on HiFiGAN, NSF, Parallel WaveGAN, etc. Applying the above studies focused on speech synthesis as it is causes several problems in high-quality singing modeling. (high, low pitch artifacts, glitch issue, etc.) Therefore, we are trying to develop a vocoder for singing voice that combines various GAN-based vocoders for high quality while taking the pitch robustness using the source excitation signal.
Singing Voice Synthesis
Speech Processing
Creative AI
Music Production is the process of managing and overseeing the recording and production of music tracks. Intelligent Music Production (IMP) aims to introduce artificial intelligence into music production, allowing for automated processes while also reflecting the user's preferences. We believe that future studies on this topic will help artists and audio engineers simplify the complex process of traditional music production and provide them with a creative workflow.
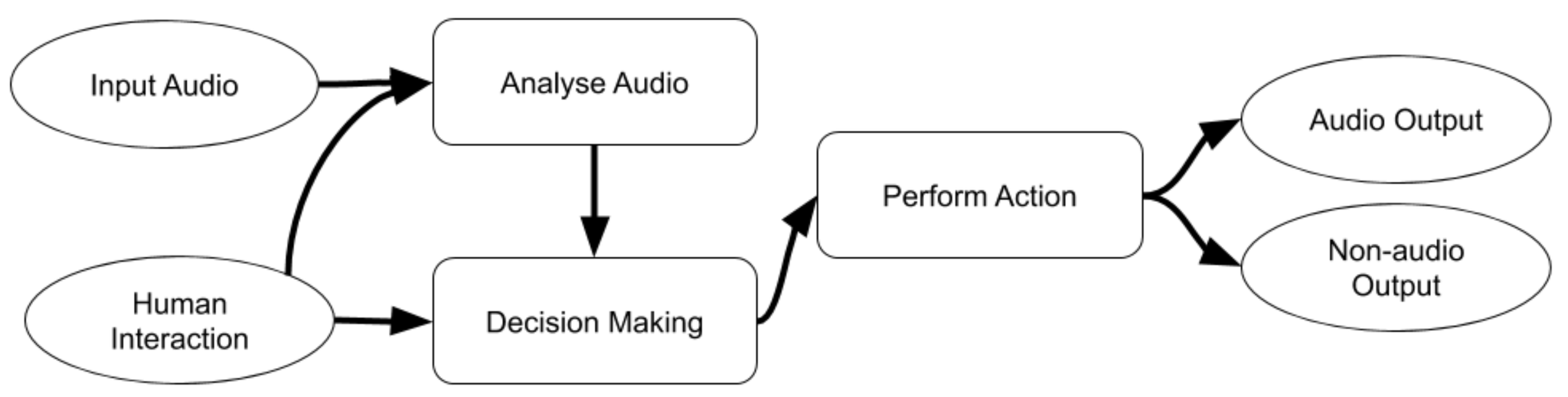
Table of Contents
Our Interests Related to IMP
Music Post Production
Music production includes various sub-processes, from transforming each audio track with audio effects to mixing and mastering. In modern music production, most recorded tracks are digitally processed so that audio engineers seek a more convenient yet intricate digital tool for their workflow. IMP should therefore introduce
1.
straightforward procedures of the overall music production,
our publication: End-to-end Music Remastering System Using Self-supervised And Adversarial Training.
2.
new digital audio effects (DAFX) that previous approaches have not been capable of,
our publication: Reverb Conversion of Mixed Vocal Tracks Using an End-to-end Convolutional Deep Neural Network.
3.
and improving the availability and accessibility of existing complex DAFX modules.
our publication: Differentiable Artificial Reverberation.
Intelligent Music Production
Signal Processing
Music Production
갤러리 보기
Search
Research Overview
Automatic music transcription (AMT) aims to extract a musical notation in a form of symbolic data from a recording of a music signal automatically. It encompasses a wide range of tasks in the music signal processing, including note onset detection, pitch estimation, and multi-instrument separation. For a full transcription, by means of the complete conversion from an audio signal to a “piano-roll” representation, two essential data of “onset/offset” and “pitch” should be obtained from both temporal and spectral analysis. In addition, our recent studies have been extended to find new features that imply musical expressions, such as a singing voice and varied playing styles of musical instruments.
Publications
•
S. Chang, K. Lee, “A pairwise approach to simultaneous onset/offset detection for singing voice using correntropy,” in Proc. IEEE Int. Conf. Acoustics, Speech and Signal Processing (ICASSP), 2014.
•
H. Heo, D. Sung, K. Lee, “Note Onset Detection based on Harmonic Cepstrum Regularity,” in Proc. IEEE Int. Conf. Multimedia and Expo (ICME), 2013.
Datasets
Project Members
Hoon Heo, Sungkyun Chang, Dooyong Sung, Yoonchang Han
Automatic Music Transcription
Transcription
Research Overview
The purpose of this research is to develop a hybrid music recommendation system that is customized to suit Korea’s music market and its music listeners. The technological advances in today’s hardware and the rapid development of the internet has lead to millions of songs being available online. However, paradoxically, there is less music for users now that more is available because of information overload. To solve this problem, music recommender systems filter out only the items that are relevant and of value to the user.
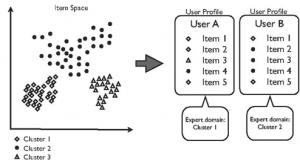
Figure 1. Concept of the recommender system
The basic concept of the system proposed in this research is to use experts when providing recommendations to ‘novices’. A broad definition of an expert would be a person who is knowledgeable in a certain area, while a novice is one who lacks such knowledge. Thus, it is only natural that knowledge is transferred from experts to novices. In this regard, we use users who are considered experts in their domain to provide recommendations to novices in those domains.
In this recommender system, each user has a domain in which he/she is a novice and an expert. An expert is defined as a user whose item consumption is skewed, or focused, on a certain set of similar items. Likewise, a user is a novice in areas where the consumption rate is low. Taking movies as an example, it is only reasonable that a person who enjoys watching sci-fi movies can provide helpful recommendations to a user who usually watches drama but occasionally finds some sci-fi movies engaging.
Thus, in order to find experts, the items are placed in an N-dimensional space, so that similar items are placed together and dissimilar items are apart. Similar items are then clustered, which define the areas that a user can be an expert or novice in. Next, each user is analyzed to see the distribution among the clusters, or areas, that the consumed items are in and are labeled as experts for specific clusters, accordingly, as shown in Figure 1. When providing recommendations for a novice, the experts of the cluster that the user is a novice in are used to generate novel and relevant recommendations.
Publications
•
K. Lee, K. Lee, Escaping your comfort zone: A graph-based recommender system for finding novel recommendations among relevant items, Expert Systems with Applications, Available online 12 August 2014, ISSN 0957-4174.
•
Z. Hyung, K. Lee, K. Lee, Music Recommendation Using Text Analysis on Song Requests to Radio Stations, Expert Systems with Applications, Volume 41, Issue 5, April 2014, Pages 2608-2618, ISSN 0957-4174.
•
K. Lee and K. Lee. Using Dynamically Promoted Experts for Music Recommendation. Multimedia, IEEE Transactions on, 16(5):1-10, 2014.
•
K. Lee and K. Lee. Using Experts Among Users for Novel Movie Recommendations. JCSE, 7(1):21-29, 2013.
Music Recommender Systems
Recommendation
Research Overview
In the last decade, there has been considerable interest in digital music services that display the lyrics of songs that are synchronized with their audio. As a recent example, Sound Hound provided a live-lyrics feature using an audio fingerprint technique, with known time stamps for alignment. Although some well-known hit songs already have lyric time stamps within existing karaoke databases, more than a hundred million numbers, including new, unpopular songs YouTube cover songs, and live recordings may not. An automatic lyrics-to-audio alignment system could reduce the huge amount of time and labor required to manually construct such time stamps. Not limited to the application above, it could also be used as a front end for various purposes in content-based music information retrieval (MIR) involving lyrical rhymes and emotions.
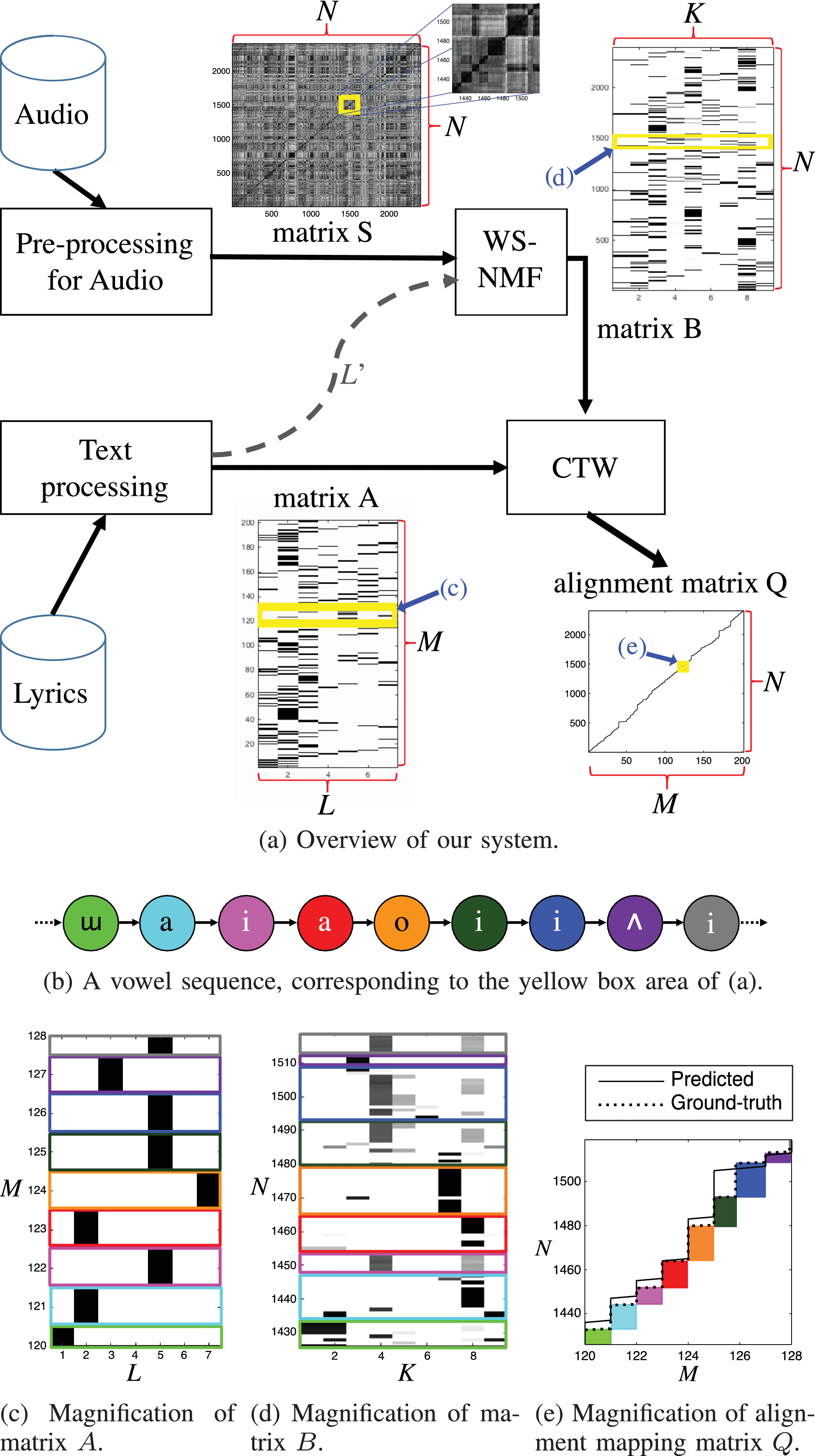
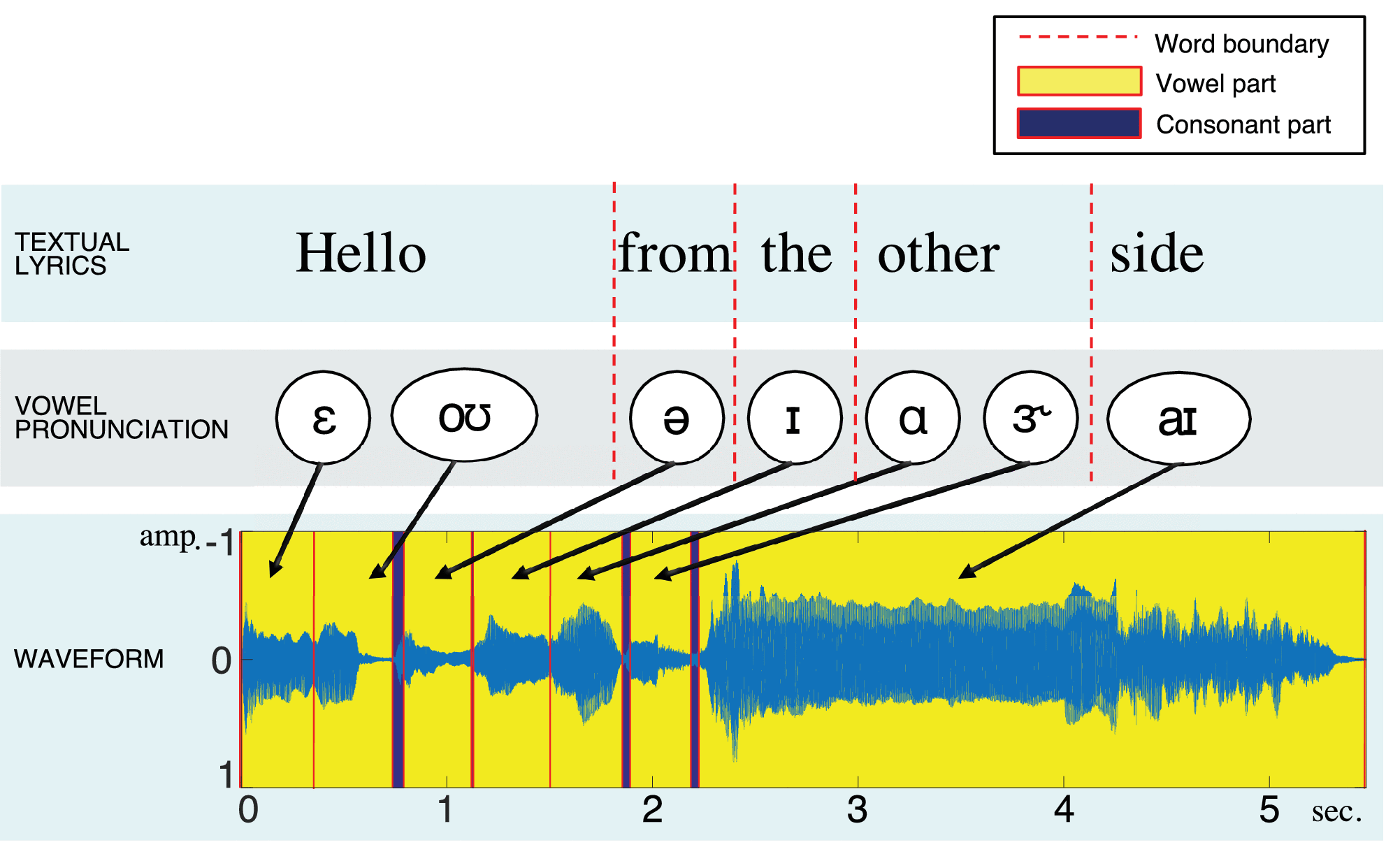
Publication
•
Sungkyun Chang, Kyogu Lee, “Lyrics-to-audio alignment by unsupervised discovery of repetitive patterns in vowel acoustics”, IEEE Access, Vol. 5, pp. 16635 – 16648, 2017
Music Lyric Alignment
Research Overview
(According to Wikipedia,) Source separation problems in digital signal processing are those in which several signals have been mixed together into a combined signal and the objective is to recover the original component signals from the combined signal. In case of music –which is combined with many musical source- we need to separate it for the better understating of it.
There are many applications which need music source separation. For example, in the music information retrieval (MIR) scene, if we want to estimate the beat of the music, we first need to obtain the percussive sound of it, while we need to remove it if we want to estimate the chord. We need to take the vocal to estimate the lyrics, and need to separate all the instruments for the music transcription.
Music source separation is not only useful for MIR, but also by itself. Let say you want to train a singing. Vocal separation can help you by providing the vocal-removed signal from the original music file. In addition, if we can set each separated source on the various virtual location, it is possible to make the upmixed version of mono->stereo or stereo->5.1ch from it.
As many other tasks, a research for the music source separation is started from specifying the problem. What do we want to separate? How many instruments are there? Is it mono, stereo, or more? Do we have any pre-trained database? Is there any other side information? Depending on the task or the application, there are numerous problems we want to solve.
Publications
Journal Articles
•
J. Park, J. Shin, and K. Lee, “Exploiting continuity/discontinuity of Basis Vectors in spectrogram decomposition for harmonic-percussive sound separation,” IEEE Transactions on Audio Speech and Language Processing. (Under review) (demo)
•
I.-Y. Jeong, and K. Lee, “Vocal separation from monaural music using temporal/spectral continuity and sparsity constraints,” IEEE Signal Processing Letters, Vol. 21, No.10, pp. 1197-1200, Jun. 2014.
Conference Papers
•
J. Park, J. Shin, and K. Lee, “Exploiting continuity/discontinuity of Basis Vectors in spectrogram decomposition for harmonic-percussive sound separation,” IEEE/ACM Transactions on Audio, Speech, and Language Processing, , Vol. 25, No. 5, pp. 1061-1074, May, 2017.
•
I.-Y. Jeong, and K. Lee, “Informed source separation from monaural music with limited binary time-frequency annotation,” submitted to IEEE International Conference on Acoustics, Speech and Signal Processing (ICASSP), Brisbane, Australia, 2015.
•
J. Park, and K. Lee, “Harmonic-percussive source separation using harmonicity and sparsity constraints,” in Proc. International Society for Music Information Retrieval Conference (ISMIR), Malaga, Spain, 2015.
Music Source Separation
Source Separation
•
Motive
•
Work in progress
Reference
[1] Losorelli, S., Nguyen, D. T., Dmochowski, J. P., & Kaneshiro, B. (n.d.). Nmed-t: A tempo-focused dataset of cortical and behavioral responses to naturalistic music. Retrieved October 13, 2021, from https://ccrma.stanford.edu/~blairbo/assets/pdf/losorelli2017ISMIR.pdf
[2] Stober, S., Sternin, A., & Grahn, A. M. O. A. J. A. (2015). TOWARDS MUSIC IMAGERY INFORMATION RETRIEVAL: INTRODUCING THE OPENMIIR DATASET OF EEG RECORDINGS FROM MUSIC PERCEPTION AND IMAGINATION. ISMIR.
[3] S. Stober, D. J. Cameron, and J. A. Grahn, “Using convolutional neural networks to recognize rhythm stimuli from electroencephalography recordings,” in Advances in Neural Information Processing Systems 27, Z. Ghahramani, M. Welling, C. Cortes, N. D. Lawrence, and K. Q. Weinberger, Eds., Curran Associates, Inc., 2014, pp. 1449– 1457.
[4] S. Stober, T. Pratzlich, and M. Muller, “Brain Beats: Tempo Extraction from EEG Data,” in Proceedings of the 17th International Society for Music Information Retrieval Conference, New York, NY, 2016.
[5] Appaji, J., & Kaneshiro, B. (n.d.). Neural tracking of simple and complex rhythms: Pilot study and dataset. Retrieved October 13, 2021, from https://ccrma.stanford.edu/~blairbo/assets/pdf/appaji2018ISMIR_LBD.pdf
[6] Cantisani, G., Trégoat, G., Essid, S., & Richard, G. (2019). MAD-EEG: an EEG dataset for decoding auditory attention to a target instrument in polyphonic music. Speech, Music and Mind (SMM), Satellite Workshop of Interspeech 2019.
[7] A. Ofner and S. Stober, “Shared Generative Representation of Auditory Concepts and EEG to Reconstruct Perceived and Imagined Music,” 19th International Society for Music Information Retrieval Conference – ISMIR 2018, pp. 392–399, 2018.
[8] Lawhern, V. J. et al. EEGNet: a compact convolutional neural network for EEG-based brain–computer interfaces. Journal of Neural Engineering 15, 056013 (2018).
If you have any questions, please contact the first author.
Reconstruction of Musical Features from EEG recordings during music listening
Neuroscience
Research Overview
The purpose of this research is to develop a real-time sound analysis technology to support unmanned surveillance systems. Industrial needs for unmanned surveillance systems are growing due to the increasing cost of labor. Today’s computer vision technology combined with deep learning algorithms is rapidly advancing to meet the goal of unmanned visual surveillance. On the other hand, auditory information is also a critical part of surveillance. Auditory information can detect abnormal events happening outside the visual angle and abnormal events which are hard to detect using visual information such as people screaming. In this research, auditory features and deep learning technology are used to achieve our objectives.
The research has three key objectives.
1. Detection and classification of abnormal sound event
2. Sound event direction of arrival(DOA) estimation
3. Robot motor noise cancellation
Publications
•
Wansoo Kim and Kyogu Lee, “Abnormal Sound Event Detection Using Auditory Transition Feature and 1D CNN”, 전자정보통신 학술대회, 대한전자공학회, 2017
•
Wansoo Kim, Gwang Seok An and Kyogu Lee, ”Detecting Abnormal Sound Events Using 4-channel Microphone Array and Deep Learning”, 음성통신 및 신호처리 학술대회,한국음향학회, 2018
•
Wansoo Kim and Kyogu Lee, “Sound Event Localization and Detection Using Modular Neural Network Structure”, 대한전자공학회 추계 학술대회, 대한전자공학회, 2018.
Real-time sound analysis technology development
Sound Detection
Research Overview
The purpose of this research is to collect respiratory sound including snoring among biological sounds generated during sleep, to be used for the development of a personalized sleep management device for the management of routine sleep in everyday life.
The research will include an analysis of sound information generated during sleep, selection of acoustic biomarker, development of a medical algorithm of a new concept based on the acoustic marker which can measure the non-invasive sleep status, development of a smart sleep mask through fusion research, and evaluation of the accuracy and efficacy of these methods using the results of sleep polyvalence test and sleep apnea test as well as a clinical trial and pilot application.
In addition, the goal of this research includes development of a smart sleep mask to a higher level, to be used for the development of various sleep-related health management scenarios using this smart sleep mask and trial application.
Publications
•
Taehoon Kim, Jeong-Whun Kim, and Kyogu Lee, “Detection of Sleep Disordered Breathing Severity Using Acoustic Biomarker and Machine Learning Techniques”, BioMedical Engineering OnLine, 17(1):16, doi: 10.1186/s12938-018-0448-x, 2018
•
Jaepil Kim, Taehoon Kim, Donmoon Lee, Jeong-Whun Kim and Kyogu Lee, “Exploiting temporal and nonstationary features in breathing sound analysis for multiple obstructive sleep apnea severity classification”, BioMedical Engineering Online, Vol. 16, No. 1, 2017
•
Jaepil Kim, Taehoon Kim, Jeong-Whun Kim, and Kyogu Lee, “An Investigation of the Snoring Sound Classification Using the Cyclostationary Attributes”, in Proc. of International Conference of the IEEE Engineering in Medicine and Biology Society (EMBC), Orlando, FL, 2016
•
Jaepil Kim, Taehoon Kim, Jeong-Whun Kim, and Kyogu Lee, “CYCLOSTATIONARITY-BASED ANALYSIS OF NOCTURNAL RESPIRATION SOUNDS”, in Proc. of International Congress on Sound and Vibration (ICSV), Athens, Greece, 2016
AcoustoSleepMask: Development of smart sleep mask using acoustic biomarker and machine learning
Research Overview
This project is supported by Urban Data Science Laboratory under the Seoul Metropolitan Government and uses big data to solve various problems in the city. In particular, the Platform part creates a platform for collecting and analyzing multiple pieces of data in the city and solves problems based on data collected by this platform.
Currently, research on real-time noise value collection and analysis in Seoul is underway, and research on real-time detection of abnormal noises that may occur in traffic and crime prevention environments in cities such as screams, glass breaking sounds, and horn sounds is underway.
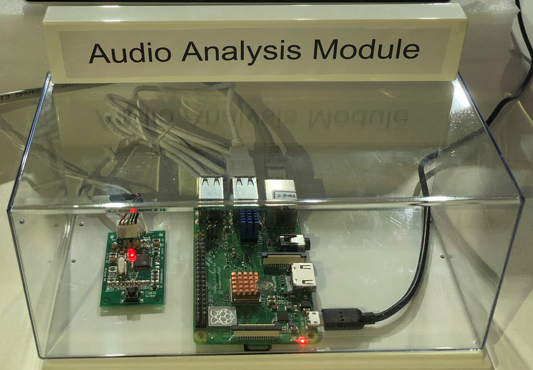
Figure 1. platform device; audio analysis module
Publications
•
Lee, Jaejun, Wansoo Kim, and Kyogu Lee. “Convolutional neural network based traffic sound classification robust to environmental noise.” (2018): 469-474.
Urban Data Science Laboratory Project for Future City with Data Foundation
Load more